The field of generative AI is swiftly advancing with the introduction of large multimodal models (LMM). These models are revolutionizing our engagement with AI by accepting both text and images as inputs. While OpenAI’s GPT-4 Vision stands out in this domain, its proprietary and commercial nature may restrict its application in some areas. Yet, the open-source realm is stepping up, with LLaVA 1.5 presenting itself as a notable open-source counterpart to GPT-4 Vision.
LLaVA 1.5 amalgamates various generative AI elements and is optimized for both computational efficiency and high precision in diverse tasks. Although it’s not the sole open-source LMM, its efficiency and performance might pave the way for future LMM studies.
Understanding LMMs
LMMs generally utilize a structure that integrates several existing components: a model for visual feature encoding, a large language model (LLM) for interpreting user commands and producing outputs, and a connector that bridges vision and language.
To train an LMM that follows instructions, a dual-phase approach is adopted. The initial phase aligns visual aspects with the language model’s word space using image-text pairs. The subsequent phase, which focuses on visual instruction, is often intricate due to its computational demands and the requirement for a vast, meticulously assembled dataset.
LLaVA’s Efficiency Explained
LLaVA 1.5 employs OpenAI’s 2021 CLIP model for visual encoding, which was designed to link images and text. This model has been instrumental in advanced models like DALL-E 2. LLaVA’s linguistic model, Vicuna, is a refined version of Meta’s open-source LLaMA model tailored for instruction adherence.
The foundational LLaVA model leveraged text-only versions of ChatGPT and GPT-4 for visual fine-tuning data generation. This approach yielded 158,000 training samples for visual instructions and was highly successful. LLaVA 1.5 enhances this by integrating the language and vision models via a multi-layer perceptron (MLP) and incorporating more training data.
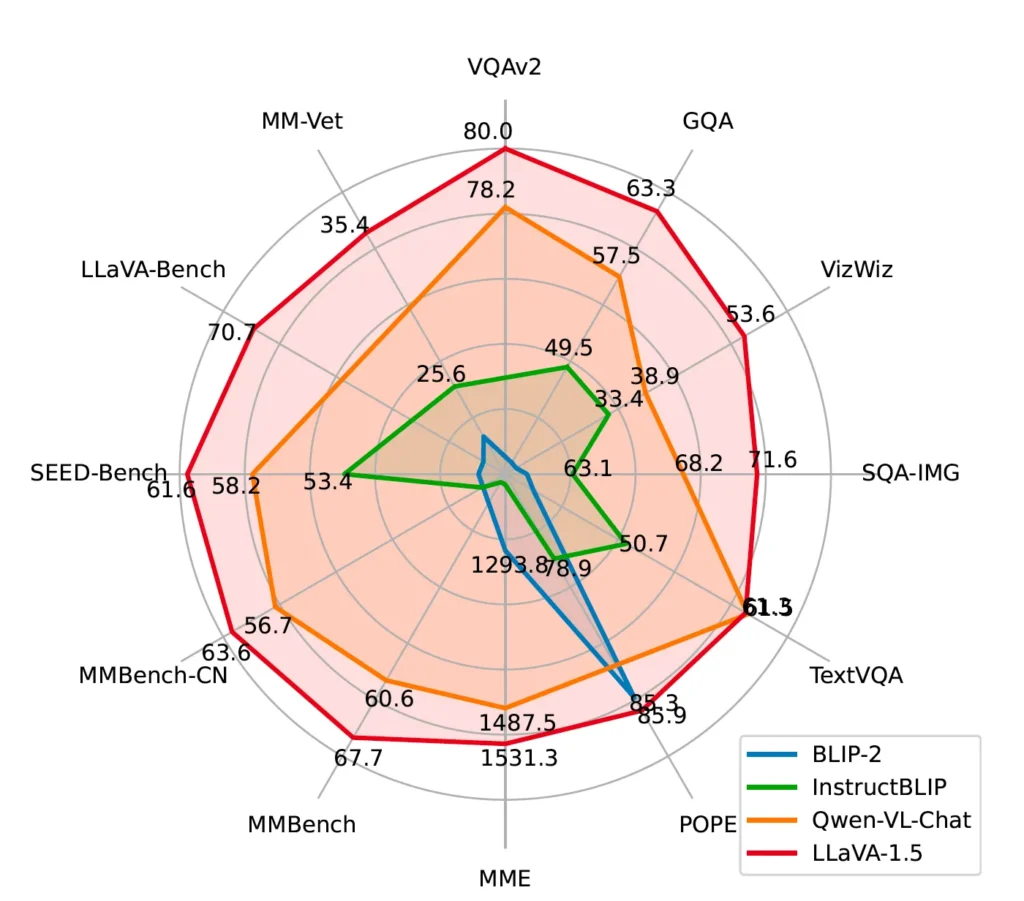
The entire dataset, comprising roughly 600,000 samples, was processed in about a day on eight A100 GPUs at a minimal cost. Research indicates that LLaVA 1.5 surpasses other open-source LMMs in most multimodal benchmarks, though gauging LMM performance can be intricate.
Open-source LLMs’ Horizon
LLaVA 1.5’s online demonstration displays remarkable outcomes from an economical model. Its code and dataset are open, spurring further advancements. Yet, due to its training on ChatGPT data, commercial utilization is restricted by ChatGPT’s terms.
While creating an AI solution has its hurdles, and LLaVA isn’t quite on par with GPT-4V in terms of convenience and integration, LLaVA 1.5’s cost-effectiveness and scalability are commendable. Several open-source alternatives to ChatGPT could be employed for visual instruction tuning, and it’s likely that the achievements of LLaVA 1.5 will be mirrored and expanded upon, including in terms of licensing and tailored models.
LLaVA 1.5 offers a sneak peek into the future of open-source LMMs. With ongoing open-source innovations, we can look forward to more streamlined and inclusive models that will broaden the horizons of generative AI.
Read related articles: